Reporting and analyzing alternative clustering solutions by employing multi-objective genetic algorithm and conducting experiments on cancer data
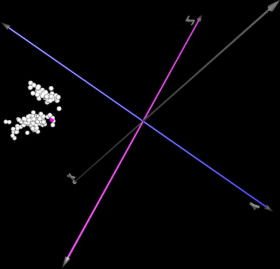
Knowledge-Based Systems 56 (2004) 108-122
Peter Penga, Omer Addama, Mohamad Elzohbia, Sibel T. Özyerb, Ahmad Elhajjc, Shang Gaoa, Yimin Liua, Tansel Özyerd, Mehmet Kayae, Mick Ridleyc, Jon Roknea, Reda Alhajja, f
Abstract
Clustering is an essential research problem which has received considerable attention in the research community for decades. It is a challenge because there is no unique solution that fits all problems and satisfies all applications. We target to get the most appropriate clustering solution for a given application domain. In other words, clustering algorithms in general need prior specification of the number of clusters, and this is hard even for domain experts to estimate especially in a dynamic environment where the data changes and/or become available incrementally. In this paper, we described and analyze the effectiveness of a robust clustering algorithm which integrates multi-objective genetic algorithm into a framework capable of producing alternative clustering solutions; it is called Multi-objective K-Means Genetic Algorithm (MOKGA). We investigate its application for clustering a variety of datasets, including microarray gene expression data. The reported results are promising. Though we concentrate on gene expression and mostly cancer data, the proposed approach is general enough and works equally to cluster other datasets as demonstrated by the two datasets Iris and Ruspini. After running MOKGA, a pareto-optimal front is obtained, and gives the optimal number of clusters as a solution set. The achieved clustering results are then analyzed and validated under several cluster validity techniques proposed in the literature. As a result, the optimal clusters are ranked for each validity index. We apply majority voting to decide on the most appropriate set of validity indexes applicable to every tested dataset. The proposed clustering approach is tested by conducting experiments using seven well cited benchmark data sets. The obtained results are compared with those reported in the literature to demonstrate the applicability and effectiveness of the proposed approach.